Extremely-Low-Power Semiconductor Devices for Sustainable AI
Professor Woo Young Choi
- Introducing extremely-low-power semiconductor devices to overcome AI limitations in terms of size, speed, and power consumption
- Proposing innovative AI architectures based on commercialized or novel semiconductor devices
Professor Woo Young Choi, from the Department of Electrical and Computer Engineering, leads the Three-Dimensional Integration and Device Lab (TIDL). TIDL has carried out a broad range of semiconductor device research for various applications including artificial intelligence (AI). Innovative neural networks (NNs) and content addressable memories (CAMs) are implemented by using commercialized (VNAND, DRAM, SRAM) memory or novel semiconductor devices (ferroelectric synaptic devices, charge-trapped synaptic devices, tunnel FETs, nanoelectromechanical devices, and so on)1-7.
Among them, in this article, the following topic will be discussed: one-transistor ferroelectric-based multi-bit CAM for the evaluation of the degree of data match. CAM compares all data stored in memory and the data to be retrieved at the same time based on the content, rather than sequential memory operations dependent on addresses such as random access memory (RAM). Existing RAM such as static RAM (SRAM) and dynamic RAM (DRAM) stores data during the write cycle and reads the memory stored during the read cycle. Because RAM should designate a specific memory location called an address, a sequential memory operation is inevitable. The sequential memory operation of RAM is not suitable for high-speed and large-capacity data search, which has recently become a hot topic in the fields of big data and AI. Even if research is actively ongoing to increase bandwidth, a fundamental solution is still far away. On the contrary, CAM simultaneously compares all data stored in memory and the data to be retrieved based on the content, rather than a sequential memory operation dependent on the address. Thus, it is expected that the data capacity and applicability of CAM will increase as the demand for high-speed operation becomes higher. Although the technical demand for CAM is burgeoning day by day, it has not been widely used because implementing high-capacity, low-power CAM is challenging. For example, 10 transistors are used in the case of the existing binary CAM (BCAM) and 16 transistors are used in the case of the ternary CAM (TCAM). This current technical limitation makes it difficult to implement a high-capacity CAM.
As the data capacity increases, the power consumption and operating time required for memory operation increase, which makes the capacity of CAM smaller than that of RAM. Researchers and industry workers become more interested in it as the capacity of data increases and the demand for high-speed operation increases. However, existing CAMs are not actively used due to difficulty in implementing high memory capacity and low power consumption. Nano-CAM, which uses various emerging memory devices, utilizes two or more semiconductor devices even in the case of the world's best technology level. To overcome these challenges, the researchers in TIDL implement a one-transistor (1T) ferroelectric-based multi-bit CAM for the first time in the world using ferroelectric tunnel FETs. Moreover, the 1T CAM features the degree of mismatch between input data and storage data without using processing units as well as ~100% compatibility with the current CMOS device/process technology. Thus, it is expected that commercialization will be possible with a minimum investment/period. If our proposed 1T CAM is successfully developed, it will greatly contribute to the development of high-speed, low-power, and parallel data processing technologies which are essential for the implementation of intelligent semiconductors. The results of our study can be applied to high-speed, low-power, and high-intensity parallel data processing in addition to image processing, pattern recognition, and internet routers. It can also be applied to one-shot/few-shot learning using memory-augmented neural networks (MANNs), which has recently attracted a lot of attention in the field of AI8-9.
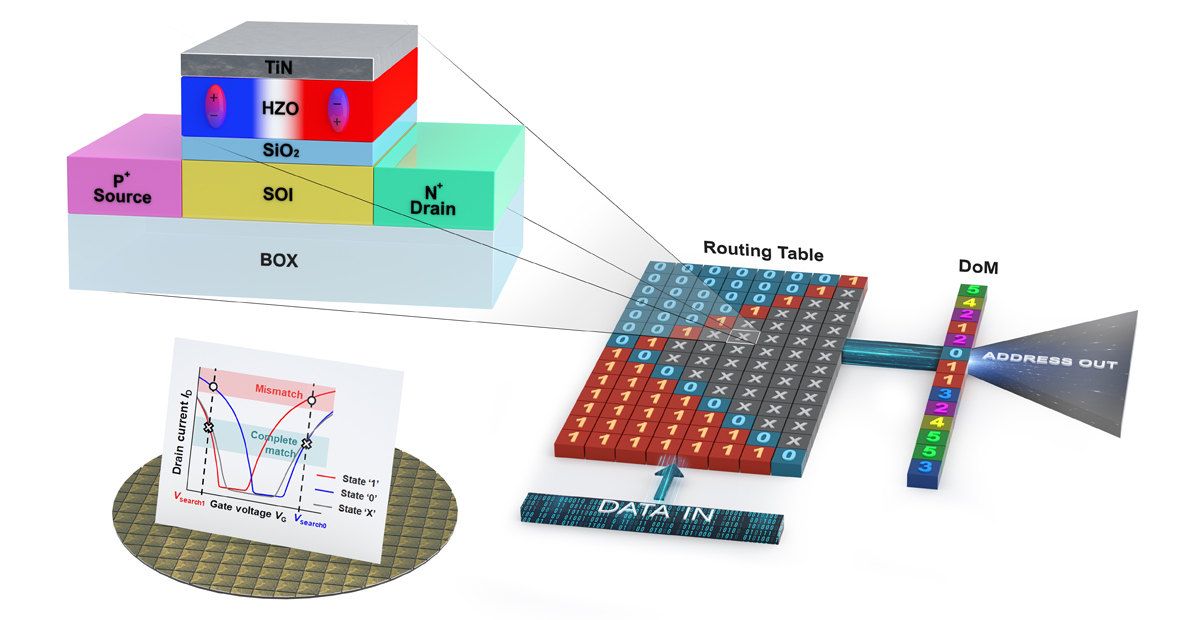
References
- 1 K. Park et al., IEEE Electron Device Letters, vol. 45, no. 3, pp. 492-495, Mar. 2024.
- 2 B. Jeon et al., IEEE Electron Device Letters, vol. 45, no. 3, pp. 340-343, Mar. 2024.
- 3 J. S. Woo et al., Advanced Intelligent Systems, p. 2300242, Nov. 2023.
- 4 T. Jang et al., IEEE Electron Device Letters, vol. 44, no. 5, pp. 833-836, May 2023.
- 5 T.-H. Kim et al., IEEE Electron Device Letters, vol. 44, no. 3, pp. 520-523, Mar. 2023.
- 6 J. Yoon et al., IEEE Electron Device Letters, vol. 43, no. 1, pp.162-165, Jan. 2022.
- 7 J. S. Lee et al., IEEE Electron Device Letters, vol. 43, no. 1, pp.154-157, Jan. 2022.
- 8 W. Y. Choi, US Patent filed 17/893,646, Aug. 23rd, 2022.
- 9 M. Ryu et al., IEEE Electron Device Letters, vol 45, no. 2, pp. 144-147, Feb. 2024.