New Memory Materials for
Energy-Efficient AI Hardware
Professor Cheol Seong Hwang
- Supporting ever-evolving computer memory
- New memristive materials for AI hardware
- Material and circuit designs for new AI algorithms
Professor Cheol Seong Hwang, from the Department of Materials Science and Engineering, leads the Dielectric Thin Film Laboratory (DTFL), where more than 90 graduate students are studying. This laboratory conducts research on two major branches of semiconductor-related materials and devices: conventional memory and logic devices (so-called Moore's law-related area) and new memristive (memory-resistor) materials for energy-efficient AI hardware.
While the former is more directly related to the semiconductor industry in Korea, so almost 40 students from Samsung Semiconductor and SK Hynix are working on it, the latter has drawn more attention from academia recently as the system scale and related energy consumption of the artificial intelligence (AI), such as large language model, have increased enormously.
Current AI systems are built on complicated software-based models, which are quite disparate from the human brain and intelligence but mostly inherited from machine learning algorithms. The most typical example is the backpropagation method to train the complex network structure composed of artificial neurons and synapses. While these methods have proven their tremendous success in diverse areas, they operate on conventional computing hardware consisting of logic circuits (arithmetic logic units) and memory, which by no means mimic the human brain, the most intelligent system. This disparity incurs fundamental issues as the AI performs human-like tasks. Also, the massive data use and transfer between the logic and memory units induce formidable energy consumption issues.
The semiconductor researchers attempt to solve such problems by creating new devices and circuits using unconventional materials like memristors. This type of research comprises a part of broader related research called neuromorphic computing (human brain-mimicking computing). A memristor is a solid-state device whose resistance depends on the voltage application or current flow history. When these devices are allocated in a matrix-type structure and connected by metal wires, they can compose a cross-bar array (CBA). CBA is a passive type of memory, which was already suggested in the 1960s, but active-type memory adopting the transistor as the cell-selection switch has dominated the field as it fits better with Moore's law.
While DTFL has focused on the CBA as a candidate for three-dimensional (3D) stacked memory, which may succeed 3D NAND flash memory in the current storage market, its recent focus is shifting toward utilizing the CBA for process-in-memory (PIM) or even neuromorphic computing1-3. PIM refers to a new computing paradigm where (part of) the computational process is performed in the memory unit, not the processors. CBA can feasibly perform multiply-and-accumulate (MAC) operations based on physical laws such as Kirchhoff's and Ohm's laws, which are well-understood in the community4. DTFL is one of the leading groups that has studied the circuit structure and material optimizations in these fields. They have recently focused on more fundamental aspects of these new computing paradigms, which may further enrich the field and eventually contribute to neuromorphic computing5-6. Among the recent achievements, they reported that the memristors could be a feasible reservoir to store time-dependent input information and project them onto a higher-dimensional feature space7-8. This capability may overcome the significant overhead problem of the convolution layer in convolutional neural network. Another notable achievement is using CBA to represent the graph, especially the non-Euclidean data, where the nodes and edges of the graphical data are represented by electrical conductance (or weight in AI terms)9-10. They figured out that the sneak current, an inherent issue of the CBA when used conventionally, can be crucial to map the correlations and interdependence between the nodes in the original graph data11. Such a new method paves ways to solve computationally intense problems, such as the traveling salesperson problem, social network evolution, human connectome analysis, and even protein-protein interconnection problems. They envision that combining these new functional computing-memory devices with smart sensors, which could also be the memristive sensors, will make the semiconductor computing system closer to the human brain.
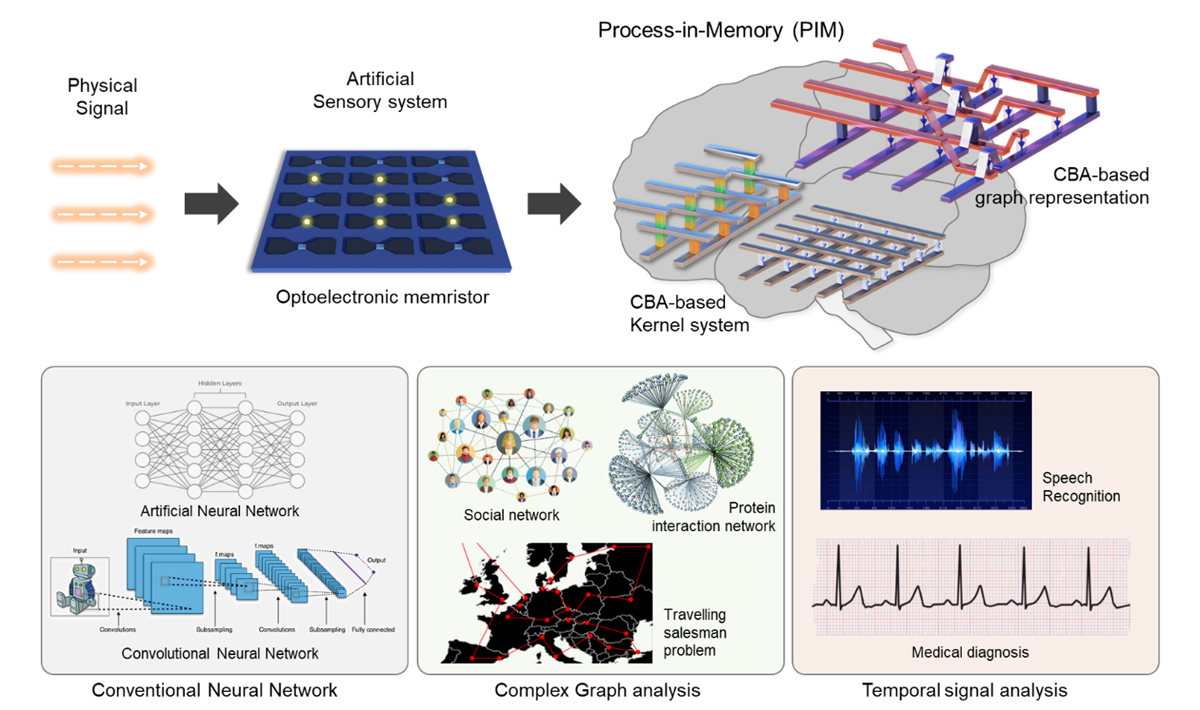
References
- 1 Kim, Seung Soo, et al., Advanced Electronic Materials, 9.3 (2023): 2200998.
- 2 Ghenzi, Nestor, et al., Nanoscale Horizons, (2024).
- 3 Jang, Yoon Ho, et al., Materials Horizons, (2024).
- 4 Cheong, Sunwoo, et al., Advanced Functional Materials, (2023): 2309108.
- 5 Park, Taegyun, et al., Advanced Intelligent Systems, 5.5 (2023): 2200341.
- 6 Park, Taegyun, et al., Nanoscale, 15.13 (2023): 6387-6395.
- 7 Jang, Yoon Ho, et al., Nature Communications, 12.1 (2021): 5727.
- 8 Shim, Sung Keun, et al., Small, (2024): 2306585.
- 9 Jang, Yoon Ho, et al., Advanced Materials, 35.10 (2023): 2209503.
- 10 Jang, Yoon Ho, et al., Advanced Materials, (2023): 2311040.
- 11 Jang, Yoon Ho, et al., Advanced Materials, (2023): 2309314.