Towards next-level autonomy: from hardware design to perception and decision making
Professor Hyoun Jin Kim
- Robot Hardware Design
- Mobile Robot Motion Control and Planning
- Perception and State Estimation
- Robot Intelligence Through Machine Learning
The Laboratory for Autonomous Robotics Research(LARR), in the Department of Aerospace Engineering at Seoul National University, led by Professor Hyoun Jin Kim, is dedicated to advancing the safe and reliable use of robots in our daily lives from both hardware and software perspectives. With a wide research spectrum ranging from environmental perception using sensors to autonomous control using actuators, LARR is recognized as one of the leading groups in robotics research.
Over the past few years, LARR has explored the future potential of aerial robots through various research, such as quadrotor drones that can access areas difficult for ground robots or workers to reach, including high-altitude tasks. The group has been conducting research on tasks like goal reaching, target tracking, cinematography, and more [Figure (a)], where one or more aerial robots carry out assigned missions while ensuring safety and leveraging their mobility in 3-dimensional space.
The lab has also been investigating the potential of aerial manipulators—aerial robots equipped with robotic arms—that can perform tasks beyond just moving. LARR's research includes both the hardware design for such systems and reliable control methods for the designed hardware [Figure (b)]. Safe and reliable control of aerial manipulators is particularly challenging since their base (the fuselage, which provides thrust) often has limited force output, and typical tasks require physical interactions with external objects (such as touching and grasping) [Figure (c)]. The lab has been devoted to developing innovative control methodologies to overcome these difficulties.
A major limitation in traditional robotics is that both hardware and decision-making software are often task-specific, making them less adaptable. Recently, machine learning methods have shown promise for handling more general, high-level tasks, such as household chores like laundry and dishwashing. LARR is working on minimizing the need for task-specific knowledge and human intervention in the robot learning process, allowing machine learning methods to be applied across various domains. To verify these learning-based decision-making methodologies, LARR uses a dual-arm robotic manipulator and a custom simulation environment designed to closely mirror real-world physics. This setup enables the seamless transfer of control strategies developed in simulation to actual robots, with the ultimate goal of creating a fully automated decision-making framework for robots, from data collection to learning [Figure (d)].
A reliable understanding of the environment, such as the positions of nearby obstacles or other robots, is critical for robots to perform intelligently and as intended. LARR conducts extensive research on using sensors like cameras and LiDAR to enhance environmental perception. This includes simultaneous localization and mapping(SLAM), which allows robots to construct maps of their surroundings and locate themselves within those maps, as well as deep learning-based computer vision techniques for robotics tasks [Figure (e)]. The lab also investigates methods to balance reliable perception and task completion, especially when these objectives conflict.
Looking toward the future of robotics utilization, LARR recognizes the importance of developing user-friendly interfaces and accessible frameworks that allow both skilled professionals and the general public to easily interact with robots. As its next research direction, the lab plans to focus on creating robot decision-making systems that can better learn from humans and engage with them using images, videos, or natural language inputs.
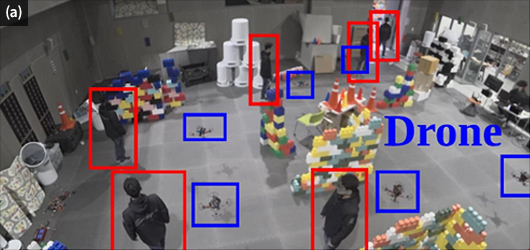
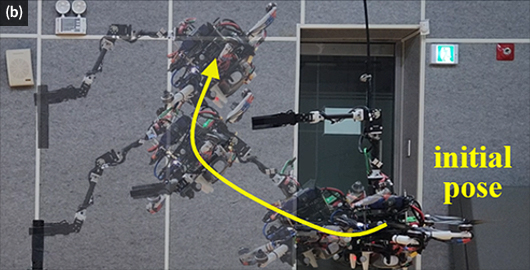
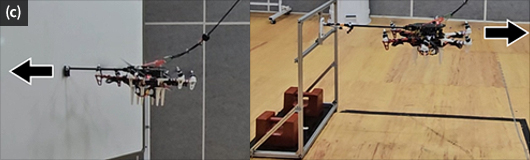
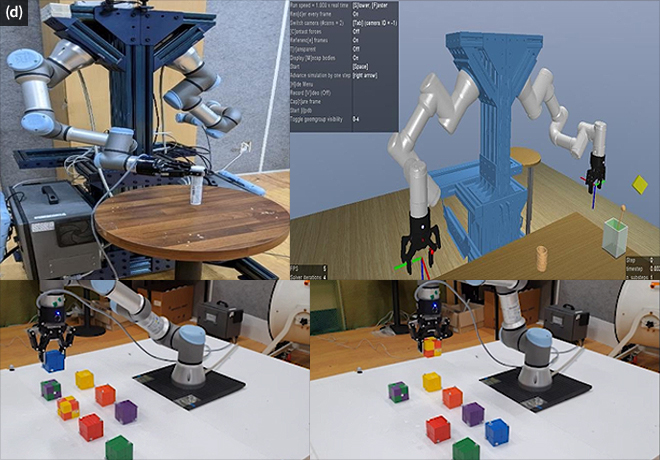
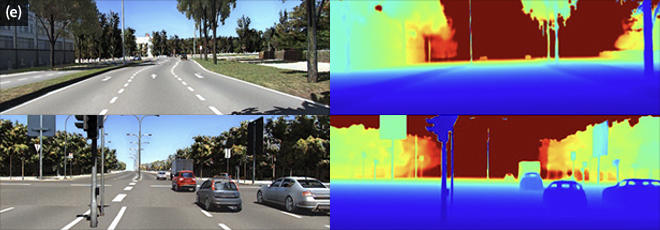
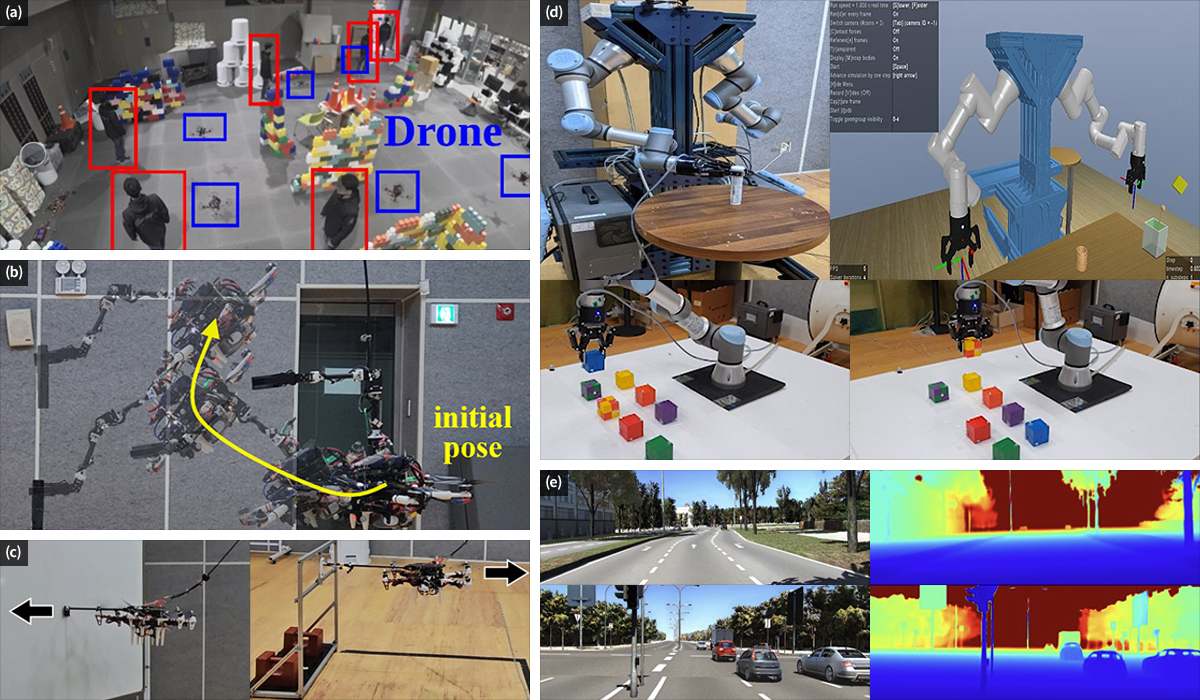