Emerging Technologies in AI-driven Autonomous Driving
Professor Jun Won Choi
Professor Jun Won Choi, from the Department of Electrical and Computer Engineering, leads the Signal Processing and Artificial Intelligence(SPA) Lab, which focuses on AI research for autonomous driving in vehicles and robots. The lab’s goal is to develop technologies that enable safe navigation in complex and dynamic environments. Their research covers two main areas: perception techniques, which extract information about the surrounding dynamic and static environments from sensor data, and planning techniques, which generate optimal and safe driving trajectories based on that information.
With recent advancements in AI, autonomous driving technology has progressed rapidly. The team believes that autonomous driving will play a key role in the future mobility revolution, significantly transforming daily commutes and the way we live. Since most vehicles remain parked for the majority of the time, autonomous driving could enable more efficient sharing of transportation resources and give people the freedom to use their time productively while in transit. Their goal is to develop autonomous driving technologies that match human-level driving performance, ensuring safe and efficient navigation even in complex environments.
However, there are several significant challenges in implementing autonomous driving technology. One of the main hurdles is the presence of numerous edge cases in real-world driving environments. Autonomous systems must be able to generalize well and handle these unpredictable situations, much like human drivers do. While humans rely on their knowledge and reasoning skills to navigate through adverse and dynamic conditions, current autonomous driving technologies still struggle to replicate these capabilities effectively.
Second, it is essential to develop planning models that can ensure a high level of safety while mimicking human driving behavior. Traditional optimization-based planning methods have difficulty adapting to new environments and often take too long to explore all possible paths in complex situations. Recently, many studies have focused on using AI models to overcome these challenges. In particular, end-to-end autonomous driving technology is emerging as a promising solution, offering more robust performance and a scalable approach to building autonomous driving systems.(see Figure.1)
Finally, new and innovative learning methods are needed for autonomous driving. Most current data from autonomous driving scenarios focus on routine lane-following situations, which offer limited value for training models in more complex or critical situations. To address this issue, learning methods must be developed that can maximize performance with minimal data. Another key challenge is generating synthetic data for critical driving scenarios. It is crucial to create high-fidelity simulations that can produce diverse and challenging scenarios, which are essential for training robust autonomous systems.
The SPA Lab is actively addressing these challenges in autonomous driving technology and pushing the boundaries of the field.
First, they are conducting research to develop robust and reliable 3D perception models capable of handling adverse and dynamic environments. Their work focuses on fusing data from cameras, radar, and LiDAR sensors to achieve robust 3D perception. They have introduced a novel camera-LiDAR fusion method in 1 and camera-radar fusion techniques in 2,3. Beyond sensor fusion, they have explored ways to enhance perception performance by incorporating temporal information. Since sensors generate continuous data streams over time, temporally fusing sequential data samples can significantly improve performance. The lab has presented two effective temporal fusion methods in 4,5. They have also worked on generating vectorized HD-MAP information using onboard sensors, as presented in 6, which they believe can contribute to autonomous driving systems that do not rely on pre-existing HD maps.(See Figure.2)
Second, the lab has developed methods to enable safer and faster planning. They have focused on improving the prediction of future trajectories for surrounding objects by leveraging static scene information and modeling interactions with other agents. This approach has significantly enhanced trajectory prediction accuracy, as demonstrated in their work 6,7. They have also released a pedestrian trajectory dataset collected from the perspective of a driving robot, which they believe will play a crucial role in future research on pedestrian movement prediction and analysis 8.(See Figure.3)
Third, the SPA Lab has been advancing machine learning techniques for autonomous driving. Active learning methods are used to select the most informative samples from the dataset, maximizing learning efficiency. Additionally, they have addressed the challenge of long-tailed data distributions, improving the robustness of models by reducing bias. Their achievements in this area have been shared in 9~11.
The SPA Lab is focused on advancing key AI technologies to ensure safe autonomous driving. Currently, they are expanding their research in perception and planning to develop fully integrated end-to-end autonomous driving systems. This approach involves directly linking perception and planning through shared features and optimizing them together. They are also exploring ways to enhance the safety of the planner by incorporating safety constraints into the neural network that determines the vehicle's decision-making. Another key research direction is leveraging vision-language models(VLMs) to improve the reasoning and generalization capabilities of the planning model, enabling better performance in diverse environments. These are timely and critical areas of research that are drawing significant attention in the field, and the SPA Lab aims to make meaningful contributions to these advancements.
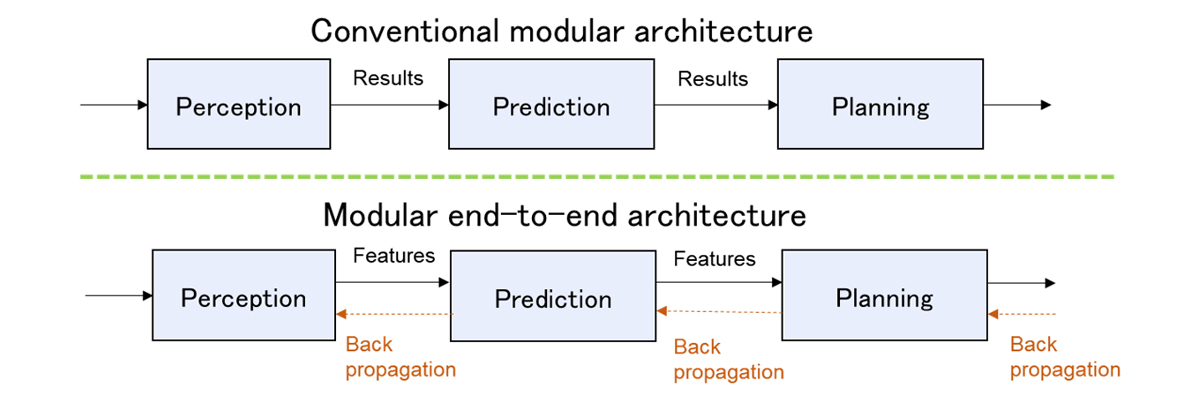
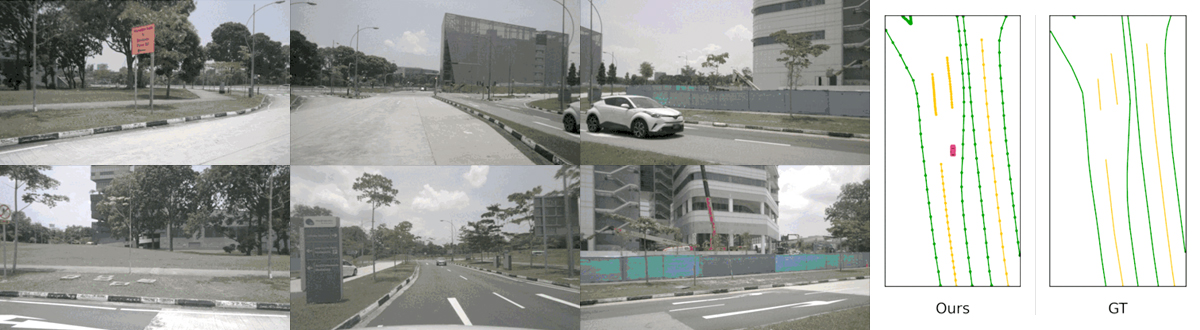
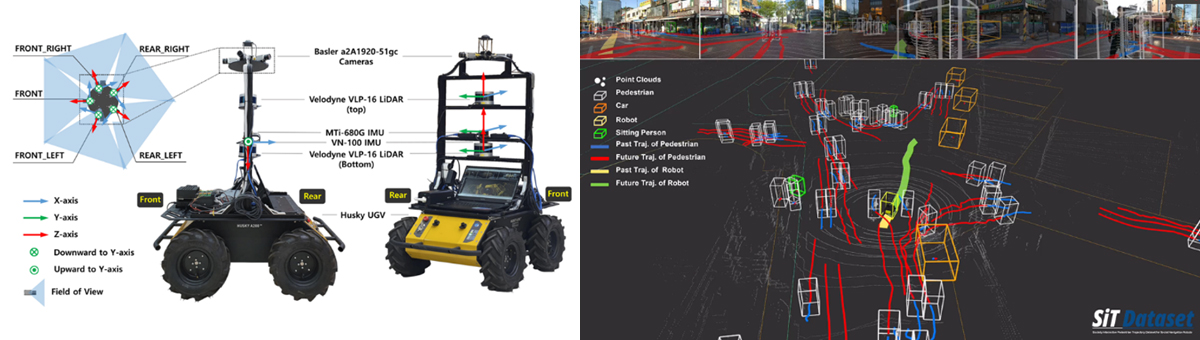
References
- 1 J. H. Yoo, Y. C. Kim, J. S. Kim, and J. W. Choi, "3D-CVF: Generating joint camera and LiDAR features using cross-view spatial feature mapping for 3D object detection," European Conference on Computer Vision (ECCV) 2020, pp. 720-736.
- 2 J. Kim, M. Seong, G. Bang, D. Kum, and J. W. Choi, "RCM-Fusion: Radar-Camera Multi-Level Fusion for 3D Object Detection," IEEE International Conference on Robotics and Automation (ICRA) 2024.
- 3 J. Kim, M. Seong, and J. W. Choi, "CRT-Fusion: Camera, Radar, Temporal Fusion Using Motion Information for Bird's Eye View Object Detection," Neural Information Processing Systems (NeurIPS) 2024.
- 4 J. Koh, J. Lee, Y. Lee, J. Kim, and J. W. Choi, "MGTANet: Encoding Sequential LiDAR Points Using Long Short-Term Motion-Guided Temporal Attention for 3D Object Detection," AAAI Conference on Artificial Intelligence 2023, 37(1), 1179-1187.
- 5 J. Lee, J. Koh, Y. Lee and J. W. Choi, "D-Align: Dual Query Co-attention Network for 3D Object Detection Based on Multi-frame Point Cloud Sequence," IEEE International Conference on Robotics and Automation (ICRA), London, UK, 2023, pp. 9238-9244.
- 6 B. Kim, S. H. Park, S. Lee, E. Khoshimjonov, D. Kum, J. Kim, J. S. Kim, and J. W. Choi, "LaPred: Lane-Aware Prediction of Multi-Modal Future Trajectories of Dynamic Agents," IEEE/CVF Conference on Computer Vision and Pattern Recognition (CVPR) 2021, Nashville, TN, USA, 2021, pp. 14631-14640.
- 7 S. Choi, J. Yoon, J. Kim, and J. W. Choi*, "R-Pred: Two-Stage Motion Prediction Via Tube-Query Attention-Based Trajectory Refinement," International Conference on Computer Vision (ICCV) 2023.
- 8 J. S. Baik, I. Y. Yoon, and J. W. Choi, "ST-CoNAL: Consistency-Based Acquisition Criterion Using Temporal Self-ensemble for Active Learning," Asian Conference on Computer Vision (ACCV) 2022, pp. 3274-3290.
- 9 J. S. Baik, I. U. Yoon, and J. W. Choi, "DBN-Mix: Training Dual Branch Network Using Bilateral Mixup Augmentation for Long-tailed Visual Recognition," Pattern Recognition, vol. 147, 2024.
- 10 J. S. Baik, I. Y. Yoon, K. H. Kim, and J. W. Choi, "Distribution-Aware Robust Learning from Long-Tailed Data with Noisy Labels," European Conference on Computer Vision (ECCV) 2024.