Transparent Object Perception and Manipulation
Professor Ayoung Kim
- Transparent Object Perception
- Transparent Object Manipulation
- Transparent Object Grasping
Professor Ayoung Kim leads the Robust Perception and Mobile Robotics(RPM Robotics) Lab at Seoul National University, where her research focuses on developing spatial perception systems for robotic applications. A key area of interest is object manipulation, a vital task for robots in various industries. To successfully grasp and manipulate objects, robots must accomplish several challenging subtasks, including scene understanding, object detection, pose estimation, and precise control of the manipulation process.
One of the RPM Robotics Lab's specialties is the study of transparent objects—commonly found in homes, chemical laboratories, and recycling centers. Transparent materials, such as plastic and glass bottles, are ubiquitous in everyday life. However, detecting and handling these objects is a complex problem due to their sensitivity to background colors and lighting conditions, which significantly alter their appearance. Professor Kim's group is working to overcome these challenges, enhancing robotic perception in environments where transparent objects are present.
Recent works by the RPM Robotics Lab have reported a large dataset for various transparent objects. The dataset introduces TRansPose, the first large-scale multispectral dataset designed for transparent object research. It combines stereo RGB-D and thermal infrared(TIR) images, alongside object poses, to address the challenges of detecting transparent objects using conventional vision sensors. The dataset includes 99 transparent and non-transparent objects, over 333,000 images, and 4 million annotations, offering segmentation masks, ground-truth poses, and depth information. Acquired using a FLIR A65 TIR camera and two Intel RealSense RGB-D cameras, TRansPose covers diverse, real-life scenarios with various lighting conditions, clutter, and challenging setups. This work has been published in The International Journal of Robotics Research1.
Once perceived, the 6D object pose should be estimated. Prof. Kim's group introduces PrimA6D++, an ambiguity-aware 6D object pose estimation network that addresses the challenges of occlusion and symmetry in object pose prediction. Unlike conventional methods that require prior shape information, PrimA6D++ predicts object poses along with uncertainty, making it more robust in real-world scenarios. The network reconstructs three rotation axis primitive images and estimates uncertainty along each axis, which are then optimized for multi-object poses, treating the task as an object SLAM problem. The method demonstrates significant improvements on the T-LESS and YCB-Video datasets and showcases real-time scene recognition for robot manipulation tasks2.
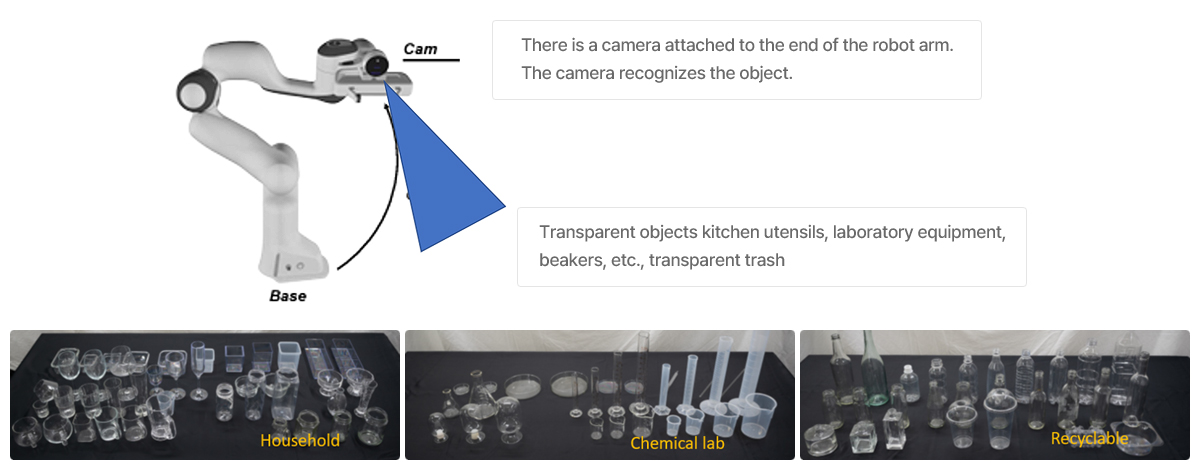
In summary, the RPM Robotics Lab, under the leadership of Professor Ayoung Kim, is making significant strides in the field of robotic perception and object manipulation. Their innovative work on transparent object detection using multispectral data, such as the TRansPose dataset, offers a comprehensive solution to the challenges posed by conventional vision sensors. By integrating thermal infrared and RGB-D data, they provide valuable insights for advancing robotic systems. Moreover, their development of the PrimA6D++ network addresses key obstacles in 6D object pose estimation, such as occlusion and symmetry, by incorporating uncertainty into the prediction process. These advancements not only contribute to more robust robotic perception but also pave the way for more practical applications in complex, real-world environments.
References
- 1 Kim et. el., IJRR, 2024
- 2 Jeon et. el., RAL, 2024