Building Reliable Robots that Learn
Professor Frank Chongwoo Park
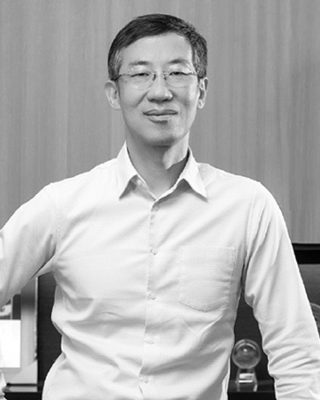
Seoul National University
To Professor Frank Chongwoo Park in the Department of Mechanical Engineering, the question of when robotics will become, like the personal computer or the mobile phone, a societally transformative technology, has been on his mind for some years now. Since robotics became an intellectual discipline and technology in its own right nearly 50 years ago, roboticists have relentlessly pursued the dream of a general-purpose robot that can competently and reliably perform tasks in home or work environments.
Advances in physical hardware -- such as motors, sensors, controllers, materials -- have made today's robots lighter, faster, more powerful, and more versatile. However, building the brain (or more precisely, the algorithms and software) that drives the robot has remained stubbornly elusive. With recent advances in AI, particularly the stunning success of large-scale foundation models in solving problems in natural language and vision that were thought unsolvable only a few years ago, roboticists are now excitedly asking whether a foundation model for robotics might be possible.
"The possibility is tantalizing and certainly should not be ruled out, but there's a long, long way to go," says Prof. Park. "Simply scaling up models and feeding them enormous amounts of data from robots will not work." He points out that data obtained from a robot is not only highly heterogeneous -- motion, force, stiffness, visual, and tactile data are just a few examples -- but also difficult and costly to collect. Simulation data obtained from physics simulators is not very reliable either, particularly for tasks that involve contact and manipulation of objects.
Robots also come in a dizzying variety of designs, topologies, and shapes: data collected from a quadruped robot is very different from data collected from an industrial manipulator or a wheeled mobile robot. Robots operate in a wide range of environments that are very different from each other. Finally, even if the data problem could be solved, he asks whether the computation and power energy requirements of foundation models really make them a feasible and sustainable way to program robots, or whether they can be made reliable and safe enough to operate in environments shared with humans.
"I believe the best way forward is the middle road," says Park. "Integrating traditional model-based methods that have gotten us this far with recent AI and machine learning-based approaches -- including foundation models developed for language and vision -- offers the best hope for deploying robots that work in the real world." Prof. Park's recent work has focused on how to merge ideas from human motor control -- movement primitives and the role of attention in learning -- with recent advances in geometric machine learning. Together with his students, he has developed neural network models that have built-in equivariance with respect to arbitrary symmetry groups and shown how these can be trained to teach robots to grasp and manipulate objects in cluttered settings. These neural network models have significantly reduced data requirements for training and generalize to a broad range of task environments. He has also developed dynamical systems-based methods for learning tasks from a single demonstration, which deliberately accommodates human-in-the-loop learning, making the process much more efficient and practically effective. How to best augment classical model-based control and planning methods with recent learning methods remains a key focus of his research.
When asked when and how the robot revolution will unfold, he replies, "It will happen quietly, I think. It's hard for me to imagine that we're going to see a robot anytime soon that everyone wants or can afford, like a mobile phone or notebook. On the other hand, we're already starting to see champions emerge in niche domains, like robots specialized for logistics, cleaning, farming, food preparation, and certain medical and healthcare applications. One day we'll look around and suddenly realize that robots have become pervasive in our lives."